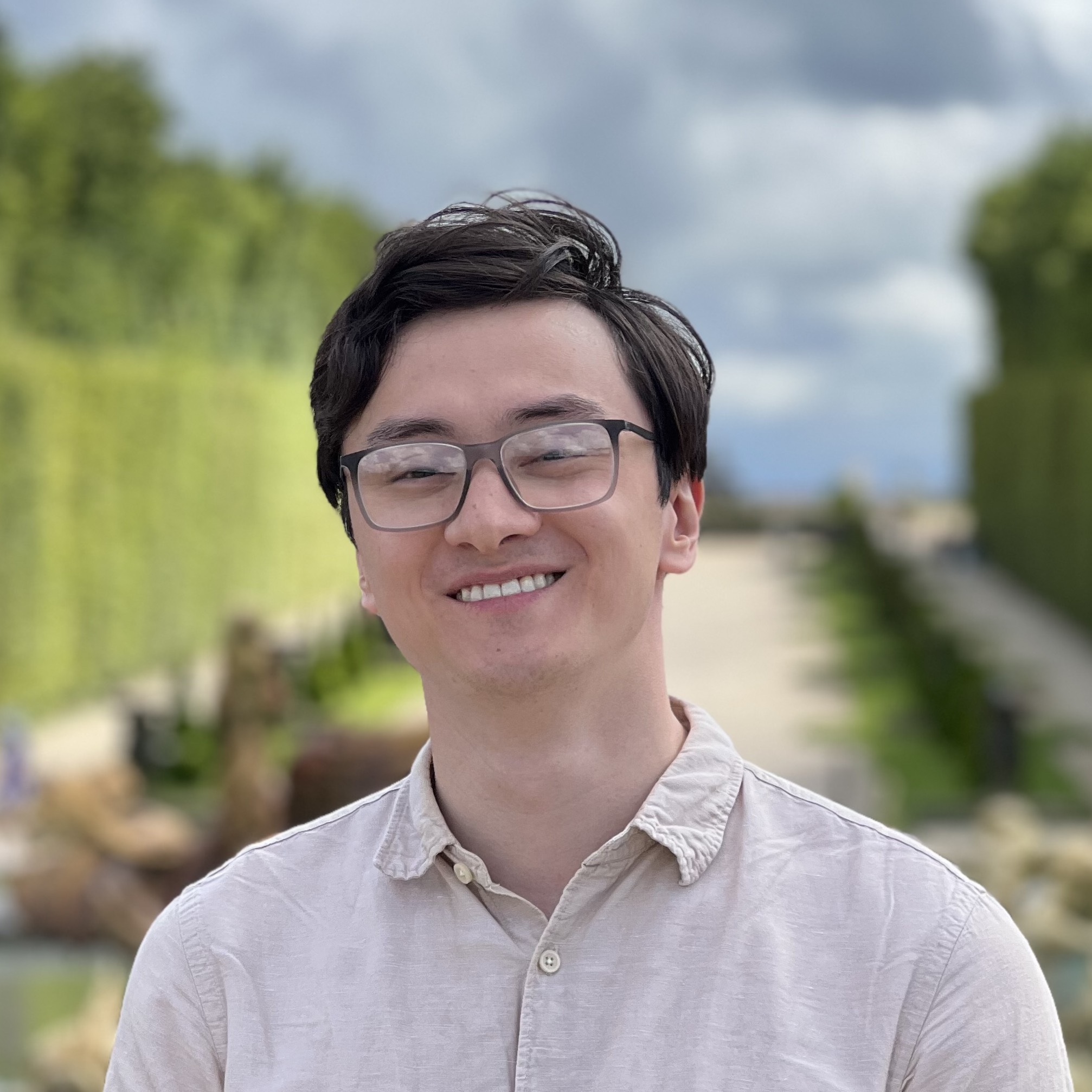
Hi! I am a PhD student at the University of Toronto working with David Duvenaud and Chris Maddison, and a research scientist at Google Deepmind. I'm interested in understanding what neural networks know, and ensuring that they act in predictable, interpretable, and safe ways in the presence of uncertainty. I'm also interested in exploring the connections between computation, intelligent behavior, and probabilistic reasoning, especially under capacity or memory constraints.
A specific direction I'm excited about is how to get powerful models to accurately report their uncertainty. Language models are surprisingly good at imitating human behavior, but frequently "hallucinate" incorrect information, making them hard to trust. How can we robustly measure the knowledge of these models, so that we know when to trust them even if we can't directly verify their reasoning? I discuss this more in my blog post on "uncertain simulators" and my recent paper "Experts Don't Cheat". I've also explored ways to summarize the uncertainty in generative models of code, described in my paper about the R-U-SURE system.
More broadly, I'm interested in methods and tools for better understanding what neural models know, how they learn it, and how they use that knowledge to make their predictions. There's so little we know about how today's models work, and I think we could learn a lot about large model behavior by studying models in controlled settings. To this end, I recently released a JAX neural network library, Penzai, that makes it easy to inspect, modify, and visualize parts of pretrained models. It's my hope that Penzai can lower the barrier of entry for research into understanding neural networks and steering them toward safe behaviors.
In the past, I have worked on generative models of discrete data structures (such as trees, sets, and graphs), theoretical analyses of self-supervised learning, a strongly-typed language (Dex) for building unconventional machine learning models, generative models for music, and many others. See my research page for more information.
I was an AI Resident at Google from 2019 to 2021, and I worked on applied machine learning at Cruise from 2018 to 2019. Before that, I was an undergraduate CS/Math joint major at Harvey Mudd College, where I did research on applying deep learning to music generation, and worked as a math tutor in the Academic Excellence tutoring program at HMC.
In my free time, I enjoy playing board games and indie video games (current recommendations: Outer Wilds, Baba is You, A Monster's Expedition, Balatro, Tunic), reading about math and programming languages, and telling myself that someday I'll get back into making music.